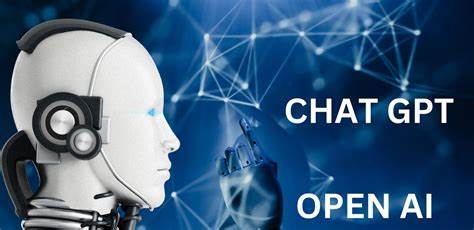
A fair evaluation of pros and cons of new Generative AI solutions called GPT Chat
This article is part of ongoing research into the impact and opportunities that Generative AI will provide for our clients.
Read more: GPT Chat: Let’s Get Down to BusinessWithout a doubt, most business leaders have heard of Chat GPT. Open AI, a Microsoft-backed artificial intelligence research firm, launched this free service in December 2022, and it quickly captured the public imagination by holding human-like conversations, writing college-level essays on any topic, generating computer code based on simple text instructions, and even passing parts of the US national medical license exam and the bar. Spend some time chatting with this online bot, and it’s difficult not to come away with the impression that this is something new and profound.
Chat GPT is one of many examples of Large Language Generative AI models, which are an evolution of earlier deep neural network natural language models. Over the last five years, these models have grown in size and power, with models now passing the Turing test – the traditional test of whether a machine can exhibit behaviour indistinguishable from that of a human.
Three factors have come together to enable this capability paradigm shift. The first was the creation of the Transformer algorithm by a Google team, which was open-sourced and quickly became the standard approach. Second, there has been an exponential increase in model size and computing power used to train these models, which now routinely cost upwards of $10M in compute time per model.
Third, these models are being exposed as simple-to-deploy APIs, allowing software developers without data science PhDs to quickly and easily develop applications that leverage their power.
While Chat GPT is receiving the majority of the attention at the moment, there is a thriving ecosystem of companies driving innovation in this space. AI experts have spun off from Google and other AI labs to form competitors to OpenAI in the text generation space, including Co:here, Anthropic, and AI21 Labs. Microsoft announced the incorporation of ChatGPT into its Bing search engine, prompting Google to respond with the release of its Bard chatbot. MidJourney, Stability.ai, and OpenAI’s DALLE-2 can generate a photo-realistic image from a simple text prompt a photo-realistic image.
But proceed with caution.
Aside from the hype, some caution is advised in the near term. ChatGPT is an impressive toy so far, but it is not a production system, with many users reporting long wait times for access and no production-level support. While OpenAI does make the underlying model available to developers via APIs for integration into production systems for a fee, there are additional reasons to exercise caution when using today’s models:
Bias and toxin – With their training data derived from the wilds of the Internet, the final model contains a lot of bias and toxic language and ideas. In a world of generative AI, responsible AI practices will become increasingly important.
Hallucination – ChatGPT can make extremely convincing-sounding arguments that are completely false. This is referred to by developers as “hallucination,” and it currently limits the ability to rely on a factual answer from these models.
Data leakage – Many companies, including Amazon, have quickly implemented policies prohibiting employees from entering sensitive information into ChatGPT for fear of it being incorporated into the model and resurfacing later in public.
Intransparency – Currently, these models provide no attribution for the facts underlying the content they generate. This makes verifying the correctness of generated claims impossible (further increasing the danger from hallucinations).
Conflicts over intellectual property – With their training data set derived from the public internet, a legal question arises as to whether the content created by these models is a copy of copyrighted works. Legal challenges have been filed, but they are still pending.
Getting better fast
However, this does not imply that Generative AI is not yet ready for prime time. OpenAI, Co:here, and Google are among the foundation model players working to quickly address these shortcomings. Look for players to take the following steps over the next six months:
Improving input data quality and output filtering – Co:careful here’s filtering of training datasets, as well as the layering of additional watchdog models on top of output, are examples of industry players’ efforts to reduce bias and toxicity. As enterprises develop applications that use their own first-party data to train or fine-tune models, the output quality for their specific use cases will improve.
Enhancing hallucination – To ensure output accuracy and address hallucination, OpenAI and other vendors are implementing measures such as data augmentation, adversarial training, improved model architectures, and human evaluation.
Adding attribution capabilities – Expect to see models incorporate attribution capabilities in the near future that can identify the original source for factual claims in output, greatly increasing end-user confidence in accuracy.
Currently, queries and actions are being incorporated. Generative models can provide answers from either their initial large training data set or smaller “fine tuning” data sets, both of which are historical snapshots. The next generation of models will understand when to look something up (for example, in a database or on Google) or when to initiate actions in external systems. This transforms the model from a disconnected oracle to a well-connected conversational interface to the outside world, opening up a slew of new possibilities.
Model vendors continue to train exponentially larger models, allowing them to make more complex conceptual connections and improve the quality of written output.
Improved efficiency – New algorithms and specialised chips will continue to evolve to improve compute efficiency.
To meet the stringent requirements of many organisations, vendors are focusing on integration with existing systems and workflows, as well as improved security and data privacy features.
To explore the usages’ of GPT Chat in current scenarios please wait for next article as conclusions….
Time to say hello to ChatGPT.